Step into a new era of pizza quality control, as we introduce our innovative solution to overcome the challenges we mentioned previously.
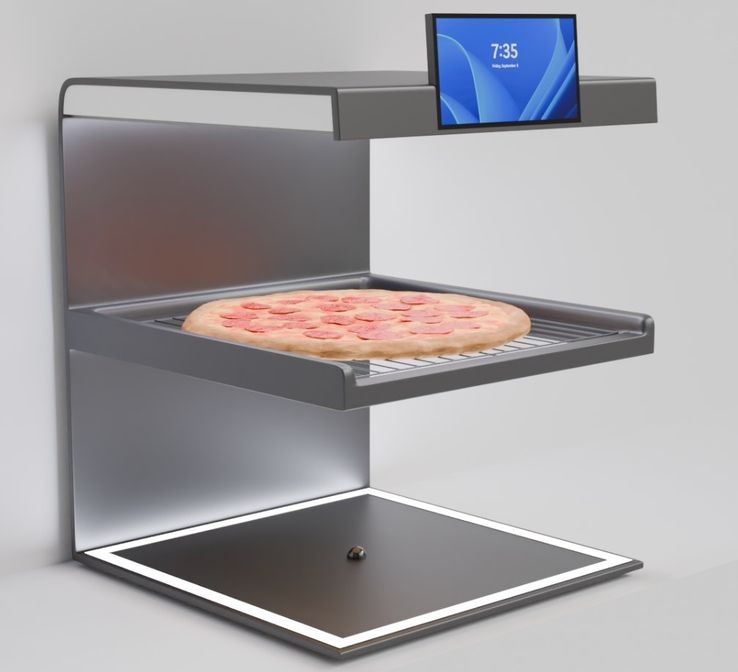 |
Pizza Quality Control Device |
After the pizza is baked, it is placed inside the QC device, the AI system starts working as soon as the two cameras start taking pictures of the pizza from both the top and bottom views. Real-time image analysis is then performed by the algorithm, which has been carefully trained on a large dataset, including a variety of crust colors and cooking phases of the pizza. The algorithm’s core functionality lies in its capacity to interpret images that indicate the doneness of the pizza.
It checks for variables like the color of the crust, how the cheese melts, and how the dough browns as a whole, examining every little aspect with unmatched accuracy. With the use of visual indicators and pre-determined criteria for well-cooked, undercooked, and overcooked pizza, the system immediately classifies the pizzas into distinct cooking quality categories using visual indicators and the mentioned preset criteria. Next, it moves on to pizza recognition by categorizing the pizza per type. Finally, the system showcases the results on an interactive embedded screen providing immediate feedback to the chef and kitchen staff presenting a clear and simple picture of the pizza quality including its doneness, shape, and topping distribution, helping the culinary team to make quicker real-time decisions whether to serve the perfected pizza or initiate a remake.
We can take as an example intelligent sensors and data analytics that are being used to guarantee freshness in food, reducing waste and increasing customer satisfaction and sales. Moreover, AI-driven systems are used in retail to ensure that products meet quality requirements and increase productivity, this digital approach to quality control also raises the bar for customer expectations, especially in the culinary world.
In the following diagram, we can clearly understand that the system detects the pizza and carefully evaluates its doneness from the top, at the same time, the second camera placed on the lower part, faces and captures the underside of the pizza.
After that, the AI does more than just locate and identify the pizza, rather, it recognizes it by giving it an identity, which is “pizza”, to accurately assess its shape (determining whether it's good or bad) and determine the appropriate distribution of toppings' based on the type of the pizza (pepperoni, rucola…).
AI Workflow Diagram |
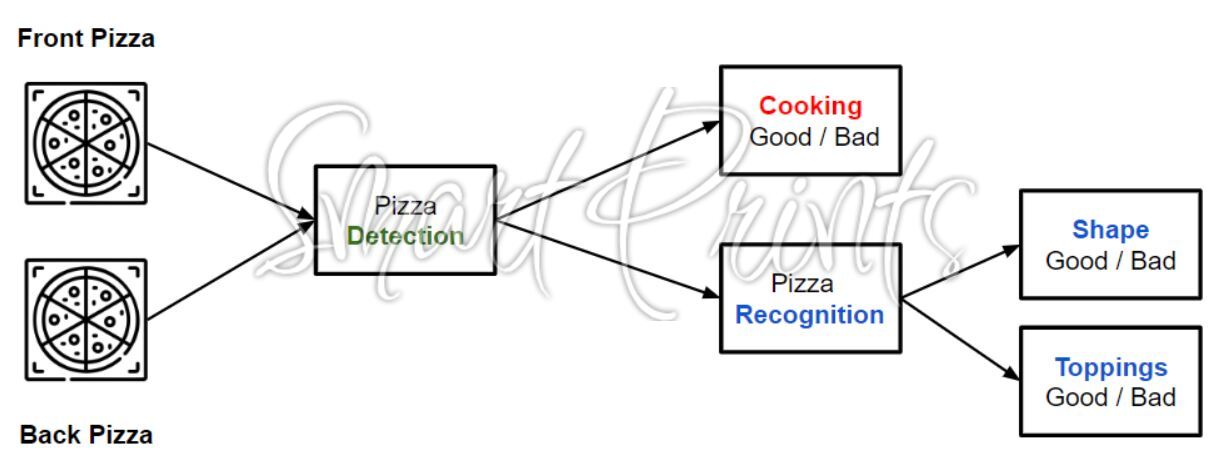 |
QC Device Hardware Specifications |
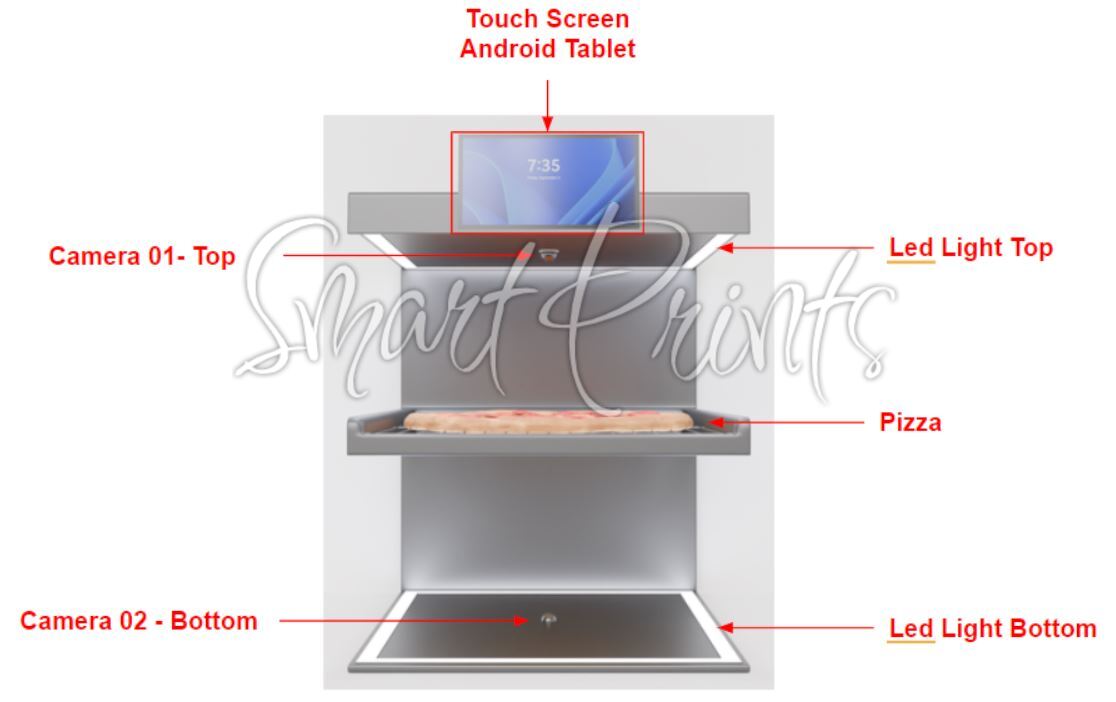 |
Built with a straightforward yet sophisticated design, our pizza quality control solution is a 50x50x60 device, featuring an interactive screen at the top facilitating seamless operation and a clear display of pizza images. The device has two strategically placed AI-powered cameras, one at the top and the other at the bottom, both cameras are set on surfaces surrounded by frames illuminated by LED lights, to ensure the best possible visibility.
Incorporated within the structure is a reserved region at the center where the pizza is placed, allowing for correct inspection from both sides. This hardware arrangement guarantees accurate and efficient quality control for every pizza.
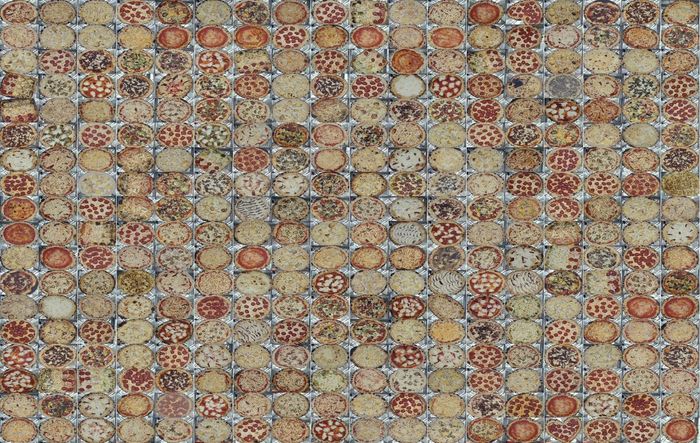 |
Dataset of 1200+ images of pizzas |
The data collection part, conducted with precision and thoughtfulness, included 1200 images of pizzas of different shapes and types, at various cooking stages (raw, baking, done), alongside some irrelevant images. These were taken in different light conditions and from various angles.
The combination of several perspectives during the data collection process facilitated the device's ability to identify and recognize various pizza characteristics.
The AI system went through robust training by being fed a diverse range of data, including multiple images of different pizza types and shapes to enable it to detect common defects like burned edges or dough, and irregular pizza shapes, and even ensure the right topping's distribution in real-time.
The system starts with a pre-processing step to enhance the image’s quality and then moves to segmentation, which aims to separate the pizza from its surroundings and background, followed by feature extraction to identify the pizza.
As for recognizing whether a pizza is well done, has the proper shape, and the right toppings, three different classifications are crucial (pizza burnt, acceptable shape, the number + distribution of toppings). Once done, and at the last stage of refinement, a post-processing procedure takes place to verify accuracy and get rid of inconsistencies.
Overview Top & Bottom |
Pizza Type Recognition |
Cooking Quality Check (0:Bad, 1:Good) |
|
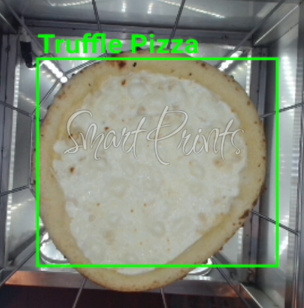 |
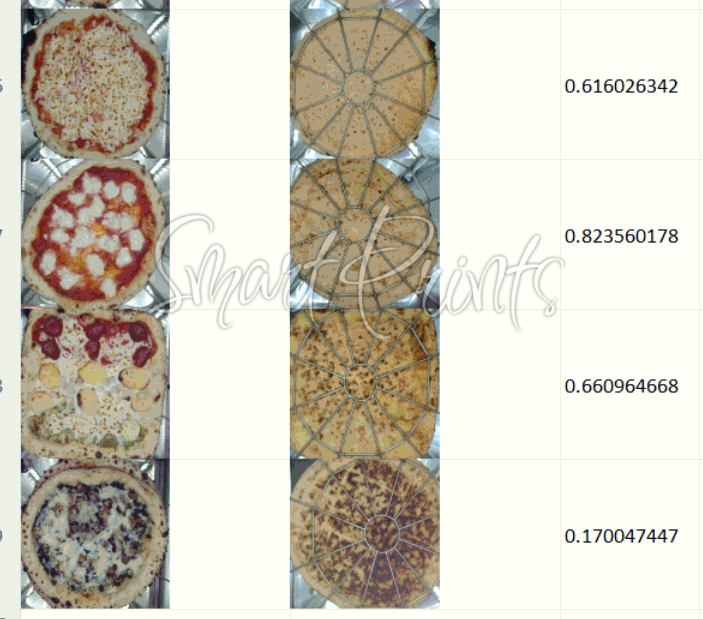 |
Results :
Conclusion :
To sum up, AI Pizza Quality Assurance has enabled LET'S PIZZA has effectively tackled the persistent problem of inconsistent pizza quality attributed to traditional wood-fired ovens. Our AI-driven Quality Control (QC) device has been implemented, successfully addressing the issue of uneven cooking.
In addition, the AI solution does more than just identify uneven cooking, it also integrates sophisticated object recognition technology allowing the system to identify different kinds of pizza by carefully counting the quantity of toppings and verifying their even distribution, guaranteeing a thorough and accurate quality evaluation to boost the gastronomic experience for customers.